Marine plastic pollution is a substantial threat to wildlife and human health. The ability to detect floating plastic debris in short periods after they enter the ocean is a mitigating factor, which avoids the degradation of the macroplastics into smaller pieces and the consequential threat to the food chain. Leveraging on the breadth of experience of the SMART consortium on deep learning, at its first stage, the SMART proposal aims at identifying different types of floating debris from satellite images and exploring the possibility to distinguish between different types of plastic. SMART is an intelligent framework based on physics-informed learning, which combines automatic identification and classification of floating plastic debris from satellite images, spatiotemporal modelling of plastic accumulations with high-resolution numerical ocean modelling and physics-guided machine learning and a distributed system of sensors mounted on low-cost marine autonomous vehicles for long-term deployment and validation of the model results. The probability of plastic occurrence map will allow authorities to devise strategies for ocean clean-up while making decisions under uncertainty. The project will be developed using two pilot sites in the North Atlantic and the results obtained will be validated in situ using low-cost marine autonomous vehicles, which will collect samples at key sensitive regions predicted by the model.
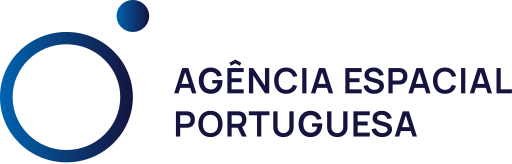